어떤 방법들이 있는지 고민해 보고 정리해 봅니다.
Method | Description | etc |
표준화된 Referece와 비교 | 글로벌 표준과 유사한 답을 내는지를 확인하는 방법 - 예로 백과사전/wikipida의 정의와 차이는 없는지? |
|
인간과 직접 비교 | - 이건 인간이 답을 알 고 있을때, 그 답을 비교 하는 것 - 예전 IBM 왓슨의 제프리 게임과 유사한 방법 |
|
인간평가자에 feedback 받기 | - AI의 답을 받고 나서, 인간이 그 답을 채점 하는 형태 | |
LLM based evaluation
- 이런 연구도 있습니다.
AI의 평가는 현재까지 사람이 한다고 생각하고 있으며, 그렇게 해 오고 있습니다. 그러나, 이걸 LLM이 AI가 직접 평가 한다는 개념의 연구 입니다.
물론 현재는 그 정확도나 신뢰성 측면에서 미비한 수준이지만, 앞으로 크게 확산되리라 생각됩니다.
- GPTScore: Evaluate as You Desire (https://arxiv.org/abs/2302.04166)
- Can Large Language Models Be an Alternative to Human Evaluation? (https://arxiv.org/abs/2305.01937)
- G-Eval: NLG Evaluation using GPT-4 with Better Human Alignment (https://arxiv.org/abs/2303.16634)
Large Language Model Evaluation Factors
When evaluating the intricacies of LLMs, a myriad of factors, ranging from technical traits to ethical nuances, need to be considered. These factors not only ensure top-notch outputs but also alignment with societal norms. Here's a succinct breakdown.
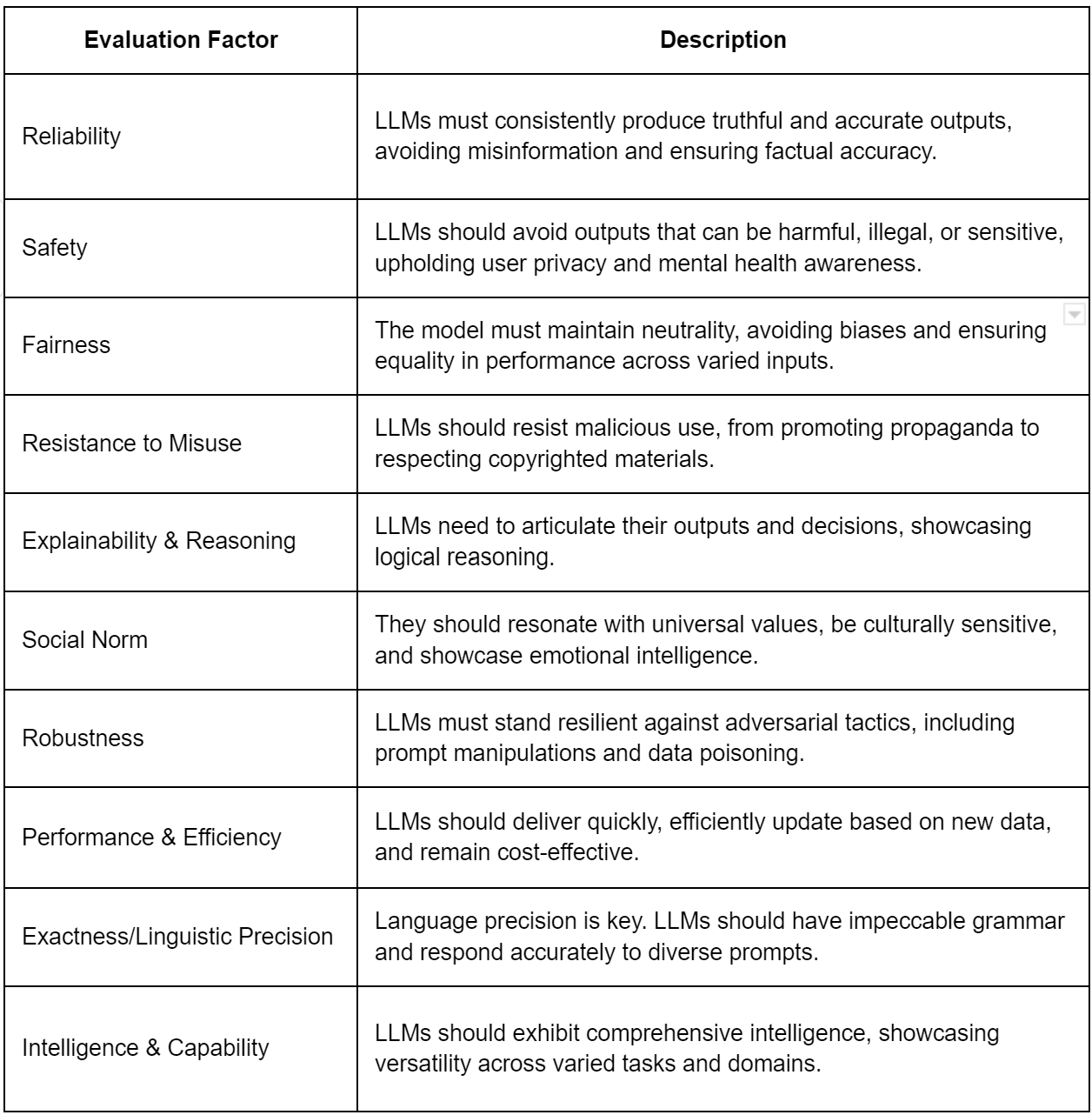
Evaluating ChatGPT as a Question Answering System: A Comprehensive Analysis and Comparison with Existing Models
In the current era, a multitude of language models has emerged to cater to user inquiries. Notably, the GPT-3.5 Turbo language model has gained substantial attention as the underlying technology for ChatGPT. Leveraging extensive parameters, this model adeptly responds to a wide range of questions. However, due to its reliance on internal knowledge, the accuracy of responses may not be absolute. This article scrutinizes ChatGPT as a Question Answering System (QAS), comparing its performance to other existing QASs. The primary focus is on evaluating ChatGPT's proficiency in extracting responses from provided paragraphs, a core QAS capability. Additionally, performance comparisons are made in scenarios without a surrounding passage. Multiple experiments, exploring response hallucination and considering question complexity, were conducted on ChatGPT. Evaluation employed well-known Question Answering (QA) datasets, including SQuAD, NewsQA, and PersianQuAD, across English and Persian languages. Metrics such as F-score, exact match, and accuracy were employed in the assessment. The study reveals that, while ChatGPT demonstrates competence as a generative model, it is less effective in question answering compared to task-specific models. Providing context improves its performance, and prompt engineering enhances precision, particularly for questions lacking explicit answers in provided paragraphs. ChatGPT excels at simpler factual questions compared to "how" and "why" question types. The evaluation highlights occurrences of hallucinations, where ChatGPT provides responses to questions without available answers in the provided context.
https://arxiv.org/abs/2312.07592
Evaluating ChatGPT as a Question Answering System: A Comprehensive Analysis and Comparison with Existing Models
In the current era, a multitude of language models has emerged to cater to user inquiries. Notably, the GPT-3.5 Turbo language model has gained substantial attention as the underlying technology for ChatGPT. Leveraging extensive parameters, this model adep
arxiv.org
'- 배움이 있는 삶 > - AI | Big data' 카테고리의 다른 글
AI Image generators (1) | 2024.01.24 |
---|---|
AI EXPO KOREA 2024 (국제인공지능대전): 24년. 5월. (0) | 2024.01.19 |
MLOps 정리 : Microsoft Azure 활용 (2) | 2023.11.29 |
AI & Big Data 관련 경진대회 (0) | 2023.07.14 |
미분 과 적분 알아보기 (0) | 2023.06.01 |